Is AI doomed to be an energy drain?
- Whether it’s training a model or making extrapolations, generative AI is energy intensive.
- The energy consumption is due to its extrapolations constantly increasing – Amazon Web Services estimates that 90% of cloud machine learning demand comes from this.
- At Télécom Paris (IP Paris), a specialised research chair is looking into how to reconcile the rise of AI with energy constraints without sacrificing its potential.
- One of the proposed solutions is to optimise models by dividing them into a multitude of “experts” capable of activating themselves according to the task at hand.
- Improving the energy efficiency of GAI would not only benefit the environment but also have a positive economic impact for those developing this type of tool.
Generative AI models, such as OpenAI’s GPT‑4, are presented as all-purpose tools. They include a significant number of parameters – now numbering in the billions – that enable them to perform any type of task. This plurality of uses, which leads to complexity issues, makes these models “in need of optimisation”, according to Enzo Tartaglione, researcher and senior lecturer at Télécom Paris (IP Paris). This complexity also implies considerable energy consumption.
“Even for an extremely simple query, AI will tend to use all the resources at its disposal to respond, without excluding those that are not useful. This leads to energy waste, and it is really something we need to optimise.” This energy consumption, estimated at around 2% of global consumption in 2024, is driving research towards an alternative approach: energy efficiency.
From training to use
OpenAI has made an extremely resource-intensive language model available via servers. This observation led researchers to distinguish between the resource consumption of model training and that of its inferences, i.e. its use. Although the energy consumption of training is significant – approximately 1,287 MWh for GPT‑3, and between 10,000 and 30,000 MWh estimated for GPT‑4 – its impact is one-off. The impact of inference, on the other hand, depends on the number of users, which is growing constantly. A 2021 study1 estimates that “between 80 and 90% of machine learning workload at NVIDIA comes from inference. Amazon Web Services estimates that 90% of cloud demand for machine learning is inference.”
Some researchers believe that a balance needs to be found between a model’s energy consumption and the task it is required to perform. If a model is used to discover a drug or advance research – which it is capable of doing – the carbon footprint will be easier to accept. However, today, these models can be used for all kinds of tasks, making millions of inferences through the various requests made of them at the same time.
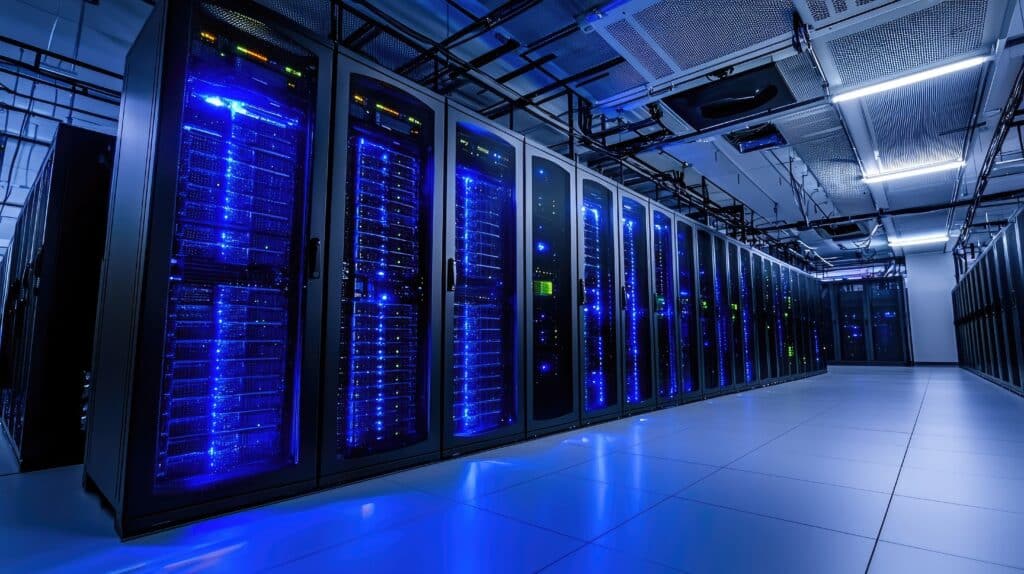
At Télécom Paris (IP Paris), the Data Science and Artificial Intelligence for Digitalised Industry and Services chair focuses on several challenges: how to reconcile the rise of AI and its energy constraints without sacrificing its potential. “We are exploring issues of frugality (editor’s note: seeking to “do more with less” and with greater respect for the environment), but also sustainability (editor’s note: meeting the needs of present generations without compromising those of future generations),” adds Enzo Tartaglione. “There is a real question in the choice of applications, because we cannot simply point the finger at AI and say it is a bad thing. Together with colleagues, we are starting work on generating materials for storing hydrogen. This is also something that AI can offer as a solution.”
Especially since the models we can all use on our mobile phones require communication with a server. “We need to be aware of the cost of transporting information, especially when it’s bidirectional,” insists the researcher. “There is therefore a great necessity to design models that can be used locally, limiting the need for communication with an external server. However, we are talking about models with billions of different parameters. This requires too much memory for your smartphone to do without the internet.”
Energy efficiency is synonymous with optimisation
There are therefore several dimensions to energy efficiency. It is not enough to reduce the number of parameters required for model calculations, whether during training or inference, as the DeepSeek model has done. Action must also be taken on the training data and the data that forms the model’s knowledge. One solution that stands out is Mistral, an open-source French language model. There is value in dividing the main model into a multitude of experts that can be activated according to the task at hand. This is one of the approaches proposed for optimising these models: distinguishing them by speciality. “The goal is to take pre-trained models and implement strategies to adapt them with as few parameters as possible to different, very specific subtasks,” explains Enzo Tartaglione. “This not only avoids the impact of retraining, but also greatly improves energy and performance.”
With more specialised models, the amount of knowledge required to achieve this will also require less data to be acquired. This type of model could therefore act locally and communicate with servers in a much more streamlined way. After all, AI is still a relatively recent innovation. And, like most technological innovations, it should logically follow the same path of optimisation as its predecessors. Ultimately, frugal AI is more of a movement in AI research than a field in its own right. In fact, computer science has always sought to design systems that optimise resources and limit unnecessary calculations – frugality is therefore a natural continuation of this logic of efficiency. Perhaps in the same way that computers and phones have become portable?
In any case, the appeal of frugality, in addition to being environmental, is also economic for the various players developing this type of tool. This does mean, however, that there is still a risk of a rebound effect: more widespread use due to lower development costs would greatly reduce the environmental benefits. However, this approach will undoubtedly not be the only solution to the energy abyss that AI represents; addressing the issue of sustainability will also be essential…