Harnessing the potential of AI for housing renovation
- AI is being used in several areas of renovation: the most promising of which today are advances are in design, to make automated plans.
- Decision-makers are faced with new variables such as climate resilience, which have no obvious technical solutions.
- At early stages of the discussion, AI can automatically generate optimised representations, which save time for decision-makers.
- Artificial neural networks can be modelled by putting them in problematic situations to develop better strategies to counter them: one will model the urban ecosystem, for example, and the other will play the role of the difficulty (cold, heat, flood).
In renovation operations, whether on the scale of a flat, a building or a neighbourhood, both energy and environmental factors are becoming increasingly important. This raises technical questions that are sometimes difficult for decision-makers to grasp. How can the use of artificial intelligence change this?
Artificial intelligence (AI) is increasingly used in renovation projects. As such, it reminds us that renovation is an area of innovation for which we can distinguish four separate areas.
The first is to classify to prioritise. AI is integrated into large-mesh diagnostic tools. For example, it will make it possible to identify – based on energy bills in relation to their size – the 1,000 or 10,000 public buildings out of 500,000, which will have the greatest impact per euro invested in terms of reducing CO2 emissions or energy consumption.
The second area is the creation of optimised renovation plans, upstream of a building site: they make it possible to improve the layout of a flat, the renovation of a building or the renewal of an urban area. The third area, at the moment of implementation, is the census. Computer visualisation techniques, which rely on images from various sources (scans, drones etc.), make it possible to take stock of everything on a site, but also to determine the percentage of progress or to identify risks.
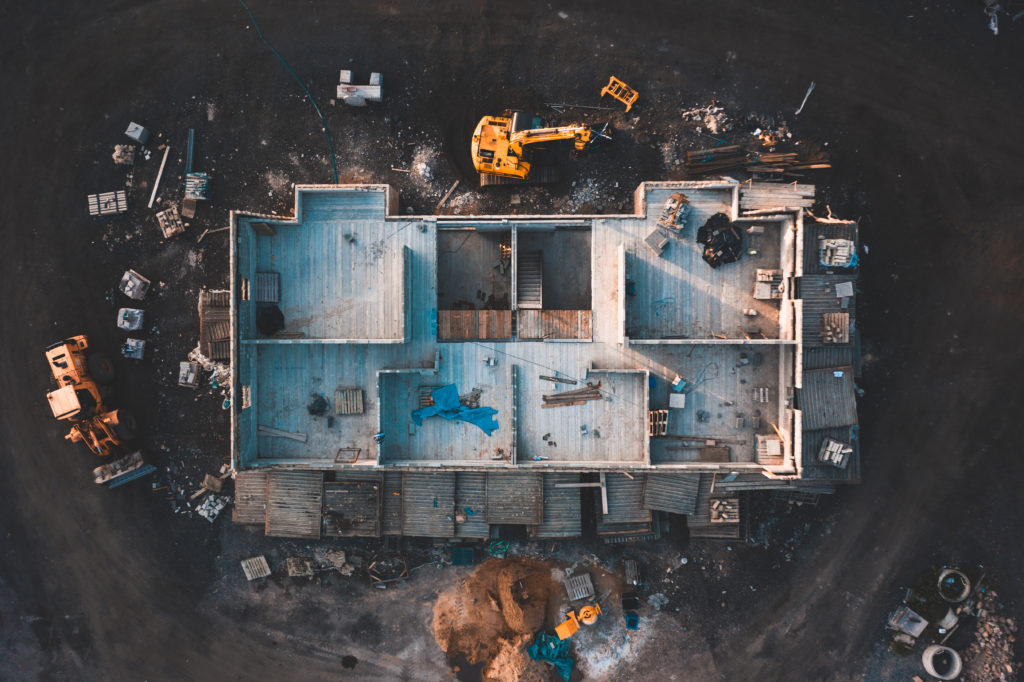
The fourth area is everything that happens when the building exists: predictive maintenance and intelligent management. Based on various sensors, AI makes it possible to anticipate breakdowns or to better understand the preferences of the building’s users. Some smart building systems go further by allowing interaction between users (or inhabitants) of the building with conversational agents, or by making predictions about the needs of residents and thus generating proposals for personalised services.
In terms of renovation, it is currently the second area, design, that is seeing the most promising advances.
With the rise of eco-design, on the one hand, and increasingly restrictive standard frameworks, on the other, this design work has become extremely complex over the last 20 years. What role does AI play in this complexity?
At the building level, technical standards are mastered by professionals and the contribution of AI is not yet major. However, it will allow for energy performance, environmental impact, or climate resilience simulations according to various objectives and constraints. This will contribute to optimising renovation solutions from an economic and environmental point of view. In a changing environment, where energy and material prices are evolving rapidly as they are now, the use of simulation allows us to reopen the field of possibilities and to find ways beyond professionals’ current habits. More generally, automation also allows work to be carried out more quickly.
On a neighbourhood scale (more rarely on the scale of an entire city), where things become more complicated, AI plays a more important role. Both for questions of resources: the industrial trades involved are practised by powerful economic players, often at the forefront of technology; and for questions of needs: it is mainly at this scale that new variables appear, which were not previously integrated and have no obvious “technical solutions”, transposed into practices.
These issues include the environmental impact of renovation activities and the resilience and adaptation of infrastructures to climate change. They are added to the more traditional ones that have governed decision-making until now: cost of the work, comfort, energy consumption in the economic sense (purchasing power of households, costs for managers), energy consumption in the ecological sense (emissions). A renovation plan is now a very complex matter!
How does AI fit in with human processes?
Basically, we are in the business of decision support. Let’s take an example. In France and in Europe, many renovation operations concern neighbourhoods or buildings belonging to the ‘social’ stock, built for the most part in the thirty years following the end of the last World War. These are therefore collective decisions, involving public actors and, of course, public money. The processes are cumbersome, the decision-makers numerous and the stakes high, because the physical deterioration of these neighbourhoods goes hand in hand with social and sometimes political problems. Moreover, they are most often heat loss areas, and the poor quality of the buildings exposes the inhabitants to energy shocks (increase in price, discomfort). Finally, the design of the neighbourhoods and buildings makes them vulnerable to the consequences of climate change, particularly heat waves and flooding. There are car parks in these neighbourhoods that are heat pockets, and those on the banks of rivers may be subject to a flood risk that was negligible when they were built.
Renovation is both an urgent and complex subject, which raises new issues that are not easy for public decision-makers to master.
To sum up, renovation is both an urgent and complex subject, which raises new issues that are not easy for public decision-makers to master. One of the essential challenges is therefore to accelerate and optimise the collective decision. This is precisely what AI allows.
How can it do so? By allowing, during the early stages of the discussion, us to automatically generate optimised representations, plans for example, which are not “the” solution, but which allow the discussion to progress. These representations are what is known in management science as “boundary objects”: they are sufficiently concrete to constitute an interface between social worlds and actors with different perspectives.
In a decision-making process that lasts a dozen months, if we take a worst-case scenario, AI could save one or two months. This is significant, without being a radical upheaval. We can also assume that the quality of the decision will be better: it is made in a way that allows everyone to understand the reasoning of others, and on the basis of representations that are easier to discuss than columns of figures. AI, by making it easy to generate proposals, is a facilitator and optimiser of human decision-making.
Can AI “take part” in the discussion?
Not in the sense that a computer would speak at the table… but a discussion dimension can be integrated into the design work that involves AI. The area that interests us in the RenovAIte R&D programme on which I am working is what is called Adversorial Resilience Learning (ARL), a concept that enables artificial neural networks to be modelled and trained by putting them in competitive situations. We take two virtual entities, which will literally throw themselves against each other. One will play the role of defender; it will be a model of the urban complex based on BIM (Building Information Modeling: digital models that do not represent geometric shapes, but objects) and other known elements. The other will play the role of the attacker: this can be any crisis (cold, heat, flooding, with risks on comfort, on heating systems, risks of cracks or impregnation, budgetary constraints, etc.). After several hours, this competition will allow each of the entities to develop the best strategies and come up with a set of optimised urban renewal plan proposals for decision-makers.
It is already working: this concept has been used in Germany in the design of smart grids, to identify malfunctions on the network and generate the appropriate automatic responses.